New estimates forecast that the global AI market will surpass $184 billion (USD) in 2024, a 35% year-over-year increase.
By all indications, AI innovation continues to increase in speed and scale. Yet new findings suggest that AI development is creating significant security concerns in cloud services. The staggering pace of AI innovation, combined with the technology’s relative nascency and an over-emphasis on speed of development, has introduced complex challenges that organizations must understand and address.
In this post, we examine the top five challenges of AI security in cloud services, why they matter, and how your organization can overcome them.
Challenge #1: Pace of innovation
The speed of AI development continues to accelerate, with AI innovations introducing features that promote ease of use over security considerations. Maintaining pace with these advancements is challenging, requiring ongoing research, development, and implementation of cutting-edge security protocols.
The AI services of cloud providers continue to expand and evolve, with new AI models, packages, and resources coming online. This year alone, for example, cloud providers each introduced a number of third-party AI models. For example, Google Vertex added models like Llama 3, Anthropic, and Mistal, while Azure OpenAI announced additions such as Whisper, GPT-4o, and GPT-4o mini. Amazon Bedrock added Cohere’s Command R models, Mistral AI, Stability AI, and more.
This rapid and steady progress presents challenges for security teams—such as properly configuring the security settings of new AI offerings (more on this later) or maintaining visibility into AI assets and risks.
Challenge #2: Shadow AI
Speaking of visibility, it often eludes security teams, especially as it relates to AI activity. Shadow AI, or the unknown and unauthorized of AI technologies in an organization, often leaves security teams with a limited view of AI risks in the cloud. Shadow AI prevents the enforcement of best practices and security policies, which in turn increases an organization’s attack surface and risk profile.
Divisions between security and development teams represent a key contributor of shadow AI. Other challenges include the lack of AI security solutions that can give security teams complete visibility into all deployed AI models, packages, and other resources in their environments, including shadow AI.
Challenge #3: Nascent technology
Due to its nascent stage, AI security lacks comprehensive resources and seasoned experts. This shouldn’t come as a surprise, considering that Google Vertex AI became generally available in May 2021, Azure OpenAI in November 2021, and Amazon Bedrock in September 2023.
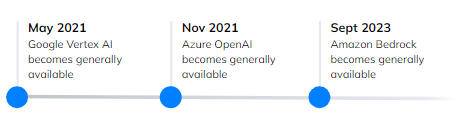
To protect AI services, organizations must often develop their own solutions to protect AI services without external guidance or examples. And while service providers offer best practices and restrictive settings for AI services, security teams may lack the time, capacity, or awareness to effectively apply them.
Challenge #4: Regulatory compliance
Navigating evolving compliance requirements requires a delicate balance between fostering innovation, ensuring security, and adhering to emerging legal standards. Businesses and policymakers must be agile and adapt to new regulations governing AI technologies. While these policies largely remain in development, we can already see their emergence with the enactment of the EU’s AI Act.
These changes to the regulatory landscape present steep challenges for organizations already struggling to achieve multi-cloud compliance. They need full visibility into their AI models, resources, and use—which remains elusive with shadow AI.
AI resources, like cloud assets, are ephemeral, spinning up and turning down at a scale and frequency that demands automation across the compliance lifecycle. This includes the following:
- inventorying AI assets, risks, and activity.
- mapping resources and risks to compliance frameworks and controls.
- continually monitoring and updating the compliance status of controls.
- resolving issues of non-compliance.
- reporting progress regularly to stakeholders.
Challenge #5: Resource control
Resource misconfigurations often accompany the rollout of a new service. Users overlook properly configuring settings related to roles, buckets, users, and other assets, which introduce significant risks to the environment.
As referenced previously, many organizations have yet to properly configure the security settings of AI services across a number of areas. This results in security risks such as exposed AI models, access keys, over-permissioned resources, and publicly exposed assets.
Solving the challenges of AI security
Fortunately, organizations can take advantage of new solutions to protect their AI innovation at scale with AI Security Posture Management (AI-SPM). AI-SPM is a type of cloud security solution that can offer security teams full visibility into AI deployments including shadow AI, advanced risk detection and prioritization, and features that automate compliance.
These capabilities depend on a native integration with a Cloud Native Application Protection Platform (CNAPP), as well as the ability for the CNAPP to provide full coverage and complete risk detection across your cloud estate.
If you’re interested in learning more about the risks of AI and recommendations for effective AI security, download your copy of Orca’s 2024 State of AI Security Report or schedule a demo with an Orca expert.